How Will StatCast Impact The Way We Value and Describe Players?
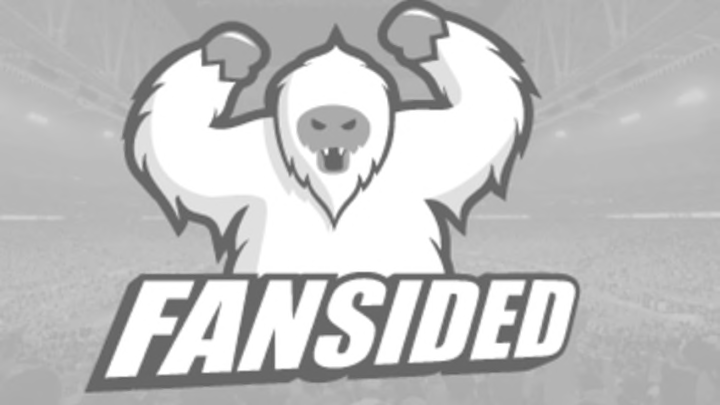
Sep 23, 2014; Cleveland, OH, USA; Cleveland Indians left fielder Michael Brantley (23) reaches for the ball on a two-RBI double by Kansas City Royals catcher Salvador Perez (not pictured) in the fifth inning at Progressive Field. Mandatory Credit: David Richard-USA TODAY Sports
Will StatCast fix all of these issues? No, it won’t. First of all: there will always be people who refuse to accept numbers that disagree with what they see. The next issue is that when dealing with fielding, we will always be dealing with a small sample of players that are actually contested. Many plays are either easy outs or hits that are impossible to catch. Let’s use Michael Brantley as an example. Inside Edge has video scouts assign an out probability for each batted ball, in buckets from 0-1%, 1-10%, 10-40%, 40-60%, 60-90%, and 90-100%. Of the 338 batted balls hit into Brantley’s vicinity in 2014 (at both center field and left field), only 28 of those balls (8.3%) had an out probability between 1% and 90%.
Some perspective on how small that sample is: that’s the same as the number of plate appearances Brantley had with a runner on first with second and third open and nobody out. You would certainly not want to judge Brantley’s batting on such a small sample, so it’s not surprising that there may be some room for error in his fielding numbers simply due to the fact that there are not that many players that are genuinely contestable.
More from Cleveland Guardians News
- Cleveland Guardians tantalizingly close to locking up AL Central tiebreakers
- Cleveland Guardians: Terry Francona becomes meme in profanity-laced ejection
- Say goodbye to defensive shifts and hello to bigger bases, pitch clock in 2023
- Cleveland Guardians: Shane Bieber second-fastest to 800 strikeouts in major-league history
- The next week will make or break the Cleveland Guardians’ season
The difficulty of distribution of out probabilities may not change when we have a better estimation of them, but it will lead to more precise valuations due to helping us understand the difference between 90% plays and 99% plays. So while our overall valuations will be better, they will still be heavily limited by the sample size. This means that a fielder who is 10 runs above average by StatCast will still have a very decent chance of having a lower true talent level than a fielder who is 5 runs above average. This is always a possibility, but it’s important to note because valuing defense is such a vital part of WAR.
With that said, while our valuation stats may only improve by a bit, our descriptive stats will become almost unimaginably better. With decent samples, we’ll basically be able to simulate a player’s chances of getting to any ball. All you need to know is: a) the hang time of the ball, b) where the ball lands, c) how quickly the fielder reacts to such a hit, d) how fast they can cover the necessary distance (taking into account initial positioning and route efficiency), and e) their chance of actually catching the ball once they get to its location. When you have all these inputs, you can then model how a fielder would theoretically perform when given a certain distribution of chances. The key to the accuracy of that model would depend on how granular the descriptive stats created and distributed publicly by StatCast will be.
The cool thing about this is that it skips the attempt to value past performance and goes directly to true talent. You’ll be able to simulate how valuable a fielder would be when playing behind different pitchers, when playing in different positions, and playing with different fielders. For example, it would make it much easier for the Kansas City Royals to determine the value of putting Jarrod Dyson into the game as a defensive replacement in the late innings, while adjusting for the pitcher, ballpark, other outfielders, and the hitters that will be batting.
This disconnect between true talent and the value of performance is going to be the most noticeable effect of StatCast’s release. I only discussed defensive metrics in this post, but hitting stats will be impacted almost as greatly. With the knowledge of how hard a player hits the ball and the angle of contact, we’ll get a much better sense of when a player has gotten lucky hits. While those lucky hits certainly led to runs and wins, they’ll look the same as outs when packaged into a model meant to estimate the player’s true talent at the plate. Again, this is going to bother some people and excite other people, but more than anything it will lead to more questions and more descriptive answers.
We may never be able to put a precise and accurate valuation on a fielder’s performance over one season. But with StatCast, we should be able to analyze their performance and understand their underlying talent in a much more interesting and evidence-based manner. All we can do now is wait for the season to start and hope that MLBAM releases granular data to the public.